Oil Sands Monitoring (OSM) Program – Leaf Area Index and Vegetation Parameters
Client:
Alberta Environment and Protected Areas
Location:
Alberta, Canada
Dates:
September 2021 – March 2023
Background:
Alberta Environment and Protected Areas (AEPA) Oil Sands Monitoring (OSM) Program sought to improve data and information on changes in vegetation parameters within the Athabasca Oil Sands area. Over the 2021-2023 period OSM required time series analysis of leaf area index (LAI), vegetation mean height (VH), and aboveground biomass (AGB) derived by remote sensing data, to provide insights into potential vegetation trends between a highly disturbed watershed and similar undisturbed baseline watershed.
In addition, OSM required monthly LAI from May to October 2022 covering the entire Athabasca Oil Sands area – a massive area of more than 16 million hectares.
The project supports the OSM program with a range of broad-scale remotely-sensed products and datasets to support watershed to regional scale monitoring of wetland vegetation changes and potential impacts from oil sands development.
Services Provided:
Priority watersheds were selected in collaboration with wetland scientists and OSM Wetland Technical Authority Committee (TAC) to scale up local surveillance monitoring to watershed scales. This selection is relative to level of oil sands activities, aiming to identify a disturbed watershed and a comparison watershed. Using remote sensing-based analytical models, vegetation health conditions and their changes over time are compared between the two watersheds, to shed light on potential impact of oil sands activities on vegetation health.
Hatfield developed and implemented the following models:
LAI – a spatially-explicit model of LAI, leveraging spaceborne optical and radar imagery, coupled with machine learning algorithms and multi-seasonal LAI field measurements. With our scalable cloud computing platform, we applied our LAI model over 16 million hectares of boreal forest monthly between May and October 2022.
VH – as an essential attribute in natural resources management, canopy height provides invaluable information about vegetation structure and forest growth. We leveraged spaceborne optical and radar data and ALS data with machine learning methods to model and map canopy height over large areas.
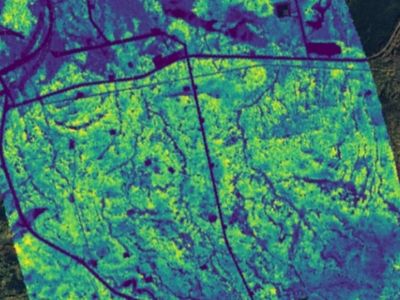