Landscape Linear Disturbance Mapping using Deep Learning Methods
Client
Canadian Space Agency
Location
Northern Alberta
Project Duration
September 2020 to March 2022
Background
Recent advances in Earth Observation (EO) technologies and the rapid expansion of advanced computing, in particular cloud computing, artificial intelligence (AI), and deep learning (DL), represent an unprecedented opportunity for Canadian Industry to develop and showcase innovative EO big data analytics. To ensure that the Canadian downstream space sector is competitive, remains at the forefront of advanced EO analytics using artificial intelligence and deep learning, and continues to be a valued international player in new emerging EO valued-added markets, the Canadian Space Agency (CSA) issued an Announcement of Opportunity (AO) entitled “Bridging the Information Gap with Space-Based Analytics – Industrial capacity-building contribution” through its newly announced smartEarth initiative.
Hatfield responded to the AO to develop a research and development project to tackle the challenge of mapping linear disturbances (i.e. amount, spatial distribution, dynamics) in the Canadian boreal forest region. Habitat fragmentation due to linear disturbances (roads, seismic exploration, pipelines, and energy transmission corridors) is a leading cause for the decline of woodland caribou (Rangifer tarandus), especially in western Canada. As a result, a deep understanding of linear disturbances (i.e. amount, spatial distribution, dynamics) has become a research and forest management priority in Canada.
Services Provided
Hatfield implemented the research project Evaluating Earth Observation Data and Deep Learning for Landscape Disturbance Mapping (EO-DL4DM).
Mapping linear disturbances from satellite imagery is important for monitoring very large areas of sensitive forest habitat like the woodland caribou range in the Canadian boreal forest region but is extremely time-consuming to complete manually through visual interpretation. Automating this process with machine learning enables a more time- and cost-effective method to cover large and remote areas. U-net inspired architectures have demonstrated potential to successfully extract paved roads in urban environments when paired with very high-resolution (< 0.5 m) imagery, but their use for general linear feature extraction in forested areas and using lower resolution imagery is not well understood. The EO-DL4DM project provided to following:
- Assessment of several deep neural networks to detect and extract landscape disturbances (primarily linear features) from satellite images;
- Trade-offs between sensor spatial/spectral resolutions to detect and extract linear disturbances;
- Demonstration of these machine learning models through pilot studies across large areas in Northern Alberta; and
- Assessment on performance and scalability using an integrated cloud computing platform.
Project findings demonstrate the potential of using UNet-inspired architectures for linear disturbance retrieval in highly dynamic forest areas. Hatfield achieved notable success in automated feature extraction from 1.5 m and 10 m satellite data, showcasing particularly strong performance in identifying roads and pipelines. Additionally, to enhance efficiency, Hatfield implemented a parallelizable cloud-based training pipeline, providing valuable insights into scalability and optimizing training time for an operational system.
Photo credit: Marc Steynen (SERNbc)
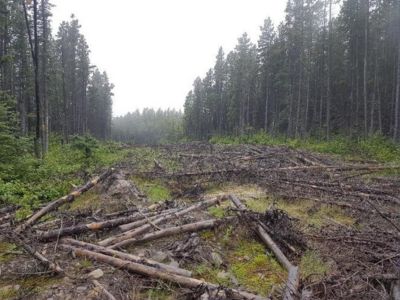